Toolkit
Experiment and brainstorm different explainable interfaces.
Scenario
Imagine you are creating an AI helper that can assist people in determining whether or not a plant is safe or poisonous. The AI helper is imperfect, therefore how might we help people determine whether to trust its prediction or use their own judgment?
In this particular instance, a person finds a blue, spotted, thorny, large plant. The AI thinks it's poisonous, but should the person trust its prediction?
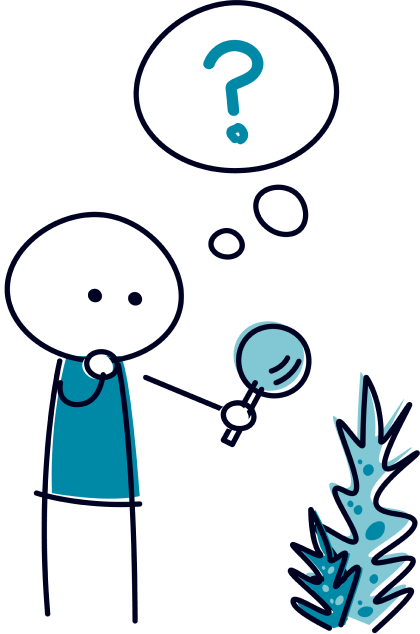
Where do I get started?
Explore how different question and explanation types can apply to this scenario by browsing and selecting a card below. Note that some explanation types may be better suited for this scenario based on the user, their goals, and the underlying model.
You can also download these cards to explore how they may be applied to your work and/or to other scenarios.
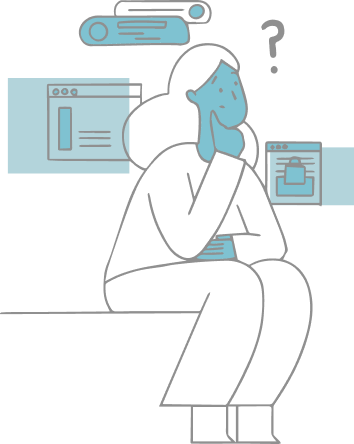
Why
Why did the system do ____?
Question example
Why did the system decide this plant is poisonous?
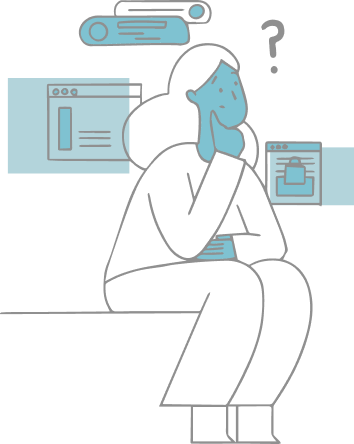
Why not
Why did the system not do ___?
Question example
Why did the system not decide this plant is safe?
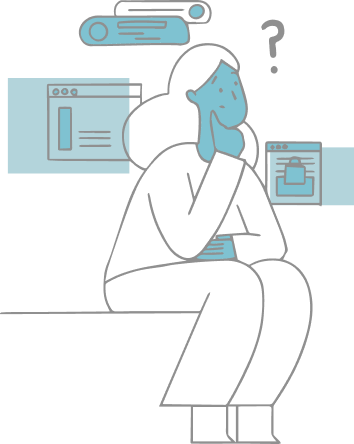
What If
What would the system do if ___ happens?
Question example
What would the system predict if the plant was smooth instead of thorny?
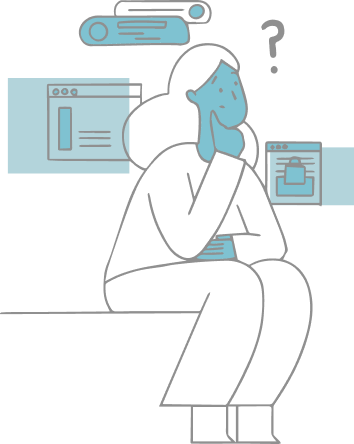
How
How (under what condition) does it do ___?
Question example
How does the system decide a plant is poisonous?
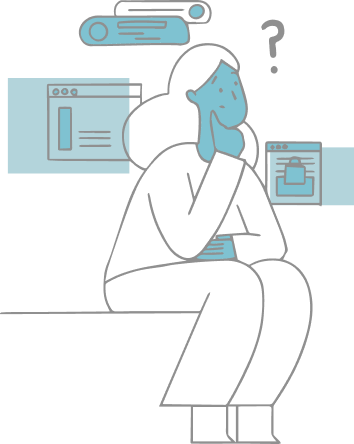
How To
Be That
What are the changes required for this instance to get a different prediction?
Question example
What would need to change for this plant to be predicted safe?
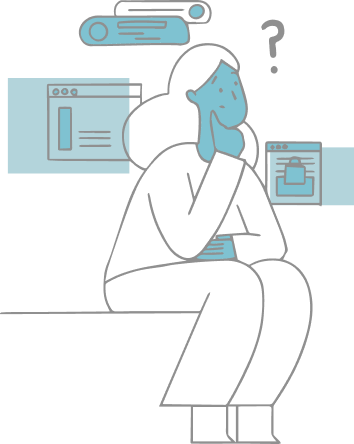
How To
Still Be This
What is the scope of change permitted to still get the same prediction?
Question example
How much would need to change for this plant to still be predicted poisonous?
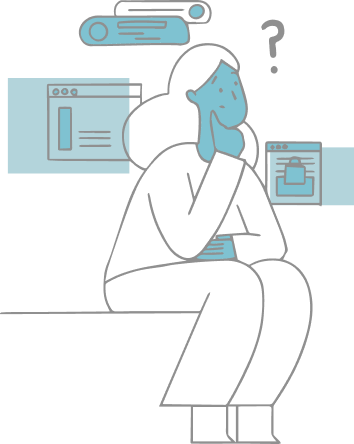
How Confident
How certain is the system in a prediction or outcome?
Question example
How certain is the system that this plant is poisonous?
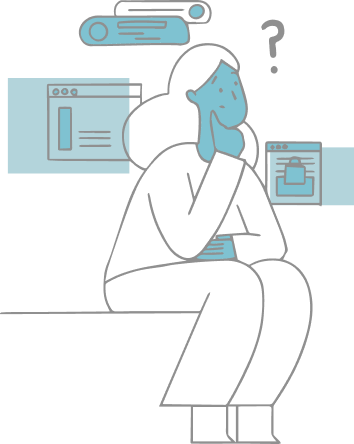
What Data
What data does the system learn from?
Question example
What information does the system use to determine whether a plant is safe or poisonous?
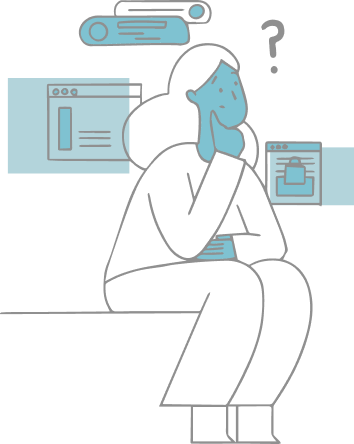
What Outputs
What are the possible outputs that the system can produce?
Question example
What can the system detect about this plant?
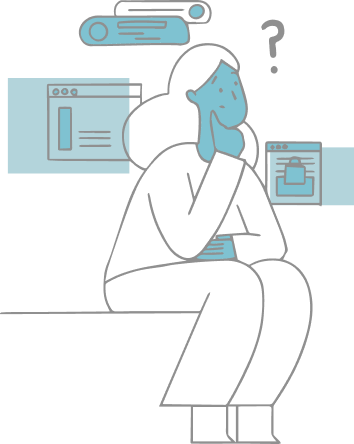
How It Works
What is the overall model of how the system works?
Question example
How does the system make its predictions?
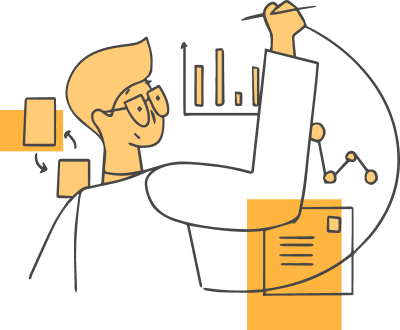
Feature Importance
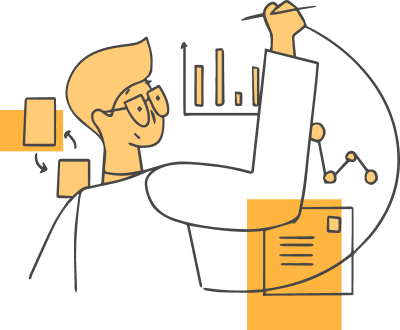
Decision Tree Approximation
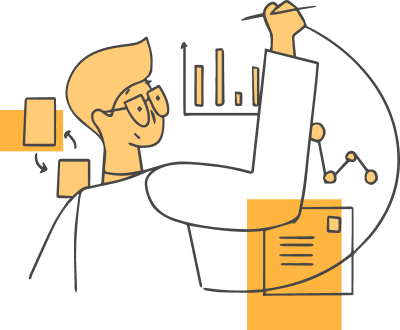
Rule
Extraction
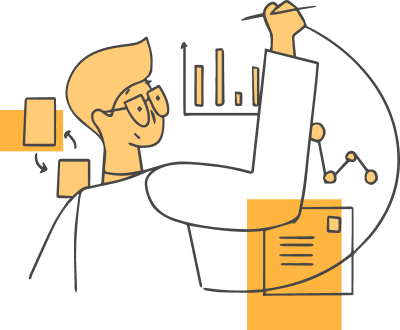
Data
Sources
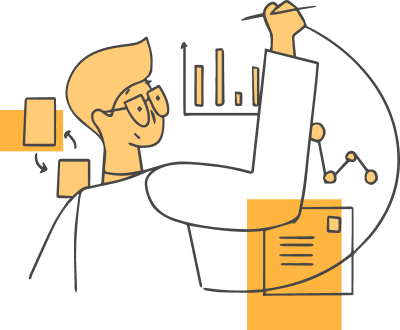
System Capabilities
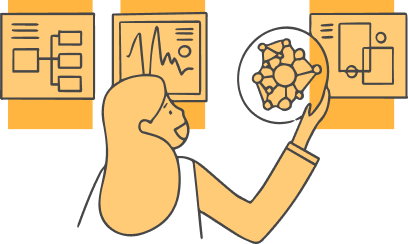
Feature Importance and Saliency
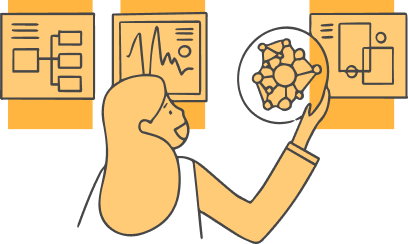
Rules or Trees
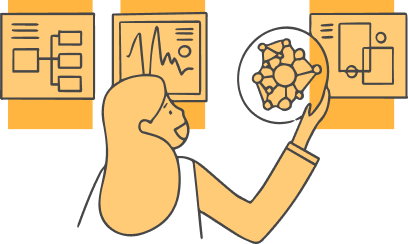
Contrastive or Counterfactual Features
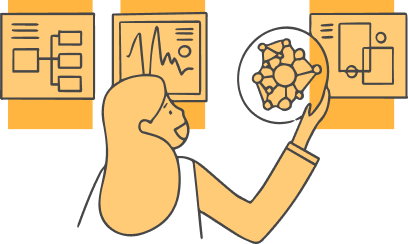
Prototypical or Representative Examples
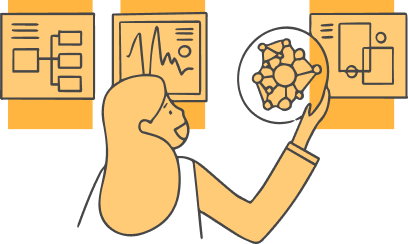
Counterfactual Example
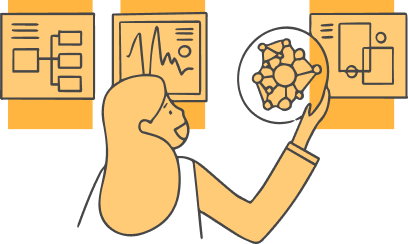
Feature Influence or Relevance
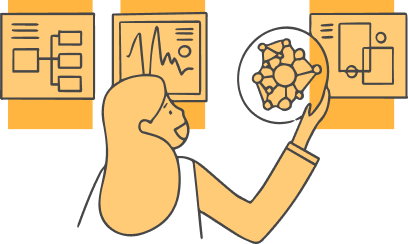
Model Confidence
References
- Google. (n.d.). People AI Guidebook.
- Liao, Q. V., Gruen, D., & Miller, S. (2020). Questioning the AI: Informing Design Practices for Explainable AI User Experiences. Proceedings of the 2020 CHI Conference on Human Factors in Computing Systems - CHI 20.
- Lim, B.Y., & Dey, A.K. (2009). Assessing demand for intelligibility in context-aware applications. Proceedings of the 11th international conference on Ubiquitous computing.
- Lim, B.Y., Dey, A.K., & Avrahami, D. (2009). Why and why not explanations improve the intelligibility of context-aware intelligent systems. CHI.
Creative Commons Attribution-NonCommercial-ShareAlike 4.0 International License